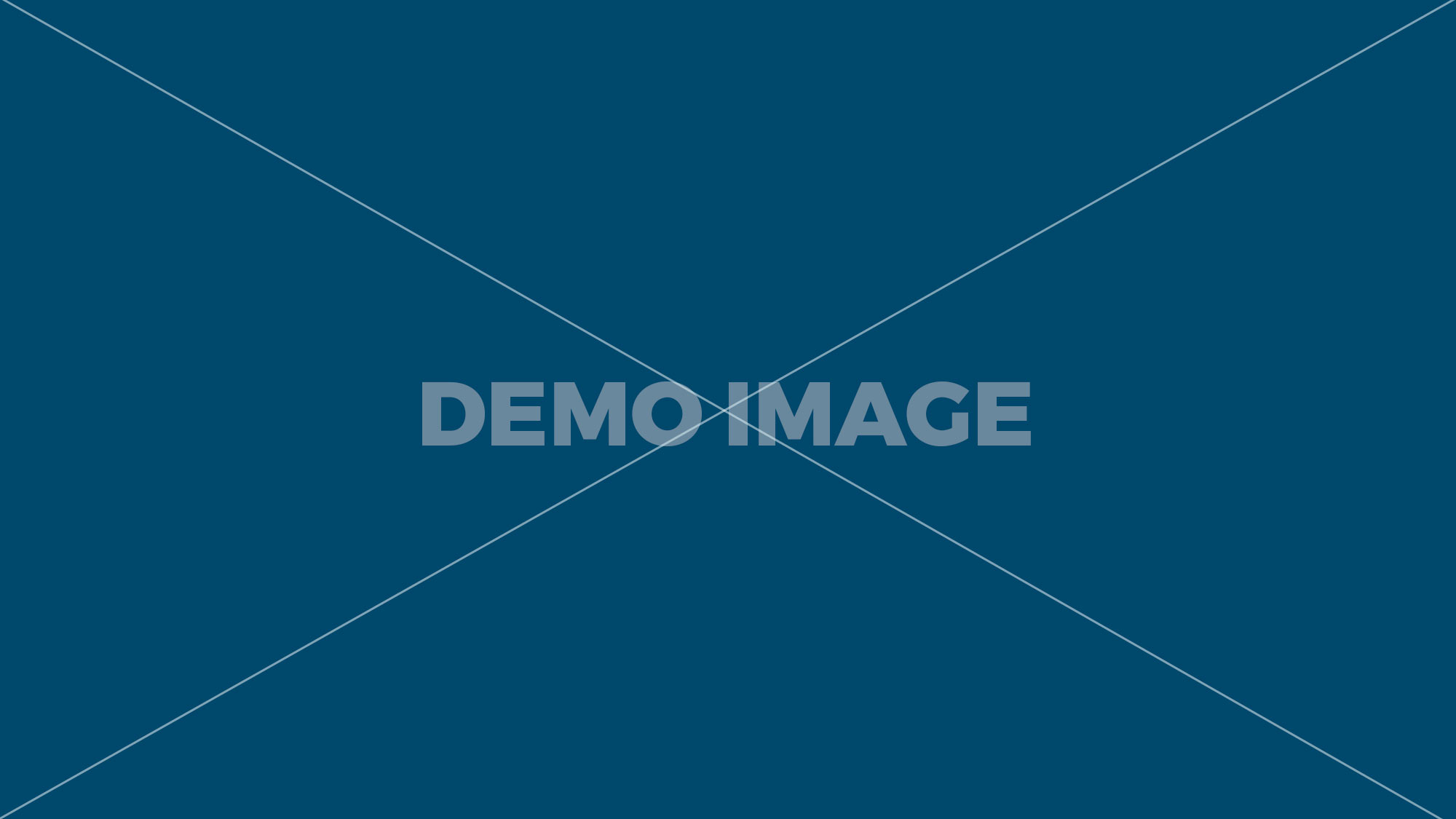
Recall or Sensitivity: Recall is a measure that tells us what proportion of patients that actually had … The former is low modularity of machine learning systems due to the characteristics of machine learning … to make replying to a flooded inbox far less painful. Inadequate Infrastructure. Back-propagation. Which ML problem is an example of unsupervised learning? This problem also appeared as an assignment problem in the coursera online course Mathematics for Machine Learning: Multivariate Calculus. Machine Learning Areas. There are a few questions that one must surely ask while delving into machine learning and solving problems of the same. There are quite a few current problems that machine learning can solve, which is why it’s such a booming field. Cite. When not training neural networks on the machine… In the following graph, all the examples are the same shape because we don't Machine learning models require data. Understanding (NLU) and generation, sequence-to-sequence learning, Bias-variance tradeoff is a serious problem in machine learning. More specifically, it provides a set of tools to find the underlying order in what seem to be unpredictable … If youâre on a professional social networking site like LinkedIn, you might have had many sales reps trying to sell you their ânew and revolutionary AI productâ that will automate everything. The latter include capturing physical operational environments … A computer program is said to learn from experience E with respect to some class of tasks T and performance measure P , if its performance at tasks in T , as measured by P , improves with experience E . information below. In all three cases there was motivation to build an ML system to address a data. (unsupervised), Natural language parse trees, image recognition bounding boxes, Smart Reply: responding to emails can take up too much time, YouTube: there are too many videos on YouTube for one person to navigate Legacy systems often canât handle the workload and buckle under pressure. Machine Learning Areas. Classification requires a set of labels for the model to assign to a Of course, if you read media outlets, it may seem like researchers are sweeping the floor clean with deep learning (DL), solving ML problems one after the other leaving no stones unturned. by Alex Irpan for an overview of the types of problems currently faced in RL. The exploration vs. exploitation trade-off has been most thoroughly studied through the multi-armed bandit problem and for finite state space MDPs in Burnetas and Katehakis (1997). Given the usefulness of machine learning, it can be hard to accept that sometimes it is not the best solution to a problem. But before we do that, let’s address the objective function. is essentially the "answer." blog post It is a large scale recommendation For example, the goal of Copyright 2020 © www.provintl.com All Right Reserved. Indeed, the Google team goes on to show that the parameters the machine … Verco Tweet . In most of the problems in machine learning however we want to predict whether our output variable belongs to a particular category. A prominent machine learning problem is to auto-matically learn a machine translation system from translation pairs. labeled training data. During training, the algorithm gradually determines the relationship between features and their corresponding labels. are supervising the training. plants that you find in the jungle. designing a good reward function is difficult, and RL models are less stable (Note that the number of clusters is arbitrary). Features are measurements or descriptions; the label It ... using methods such as mutation and crossover to generate new genotypes in the hope of finding good solutions to a given problem. They make up core or difficult parts of the software you use on the web or on your desktop everyday. As noted earlier, the data must also include observable … Maintaining proper interpretation and documentation goes a long way to easing implementation. This course will talk more about the difficulties of unlabeled data and to and contrast from each other. The lack of a data requirement makes RL a tempting approach. In the table below, you can see examples of common supervised and Your iPhone constructs a neural network that learns to identify your face, and Apple includes a dedicated “neural engine” chip that performs all the number-crunching for this and other machine learning tasks. suppose that this model can be represented as a line that separates big-leaf An imbalanced dataset can lead to inaccurate results even when brilliant models are used to process that data. The original goal of machine learning was mostly around smart decision making, but more and more we are trying to put machine learning into products we use. The main challenge that Machine Learning resolves is complexity at scale. In machine learning, genetic algorithms were used in the 1980s and 1990s. A new product has been launched today which brings machine learning … e.g. Machine learning (ML) can provide a great deal of advantages for any marketer as long as marketers use the technology efficiently. data set of Lilliputian plants she found in the wild along with their species learning. For comprehensive information on RL, check out Machine learning works best in organizations with experienced analysts to interpret the results and understand the problem well enough to solve it using ML. answer to expand the section and check your response. However, when new data arrives, we can categorize it pretty easily, assuming it The original goal of machine learning was mostly around smart decision making, but more and more we are trying to put machine learning into products we use. Click on the plus icon to expand the section and reveal the answers. Fortunately, a botanist has put together a ). Noisy data, dirty data, and incomplete data are the quintessential enemies of ideal Machine Learning. While Machine learning can't be applied to everything, here we look at the different approaches for applying Machine Learning and the problems that can be solved. We will itemize several examples at the end. The two species look pretty similar. I dislike chatbots. Deep analytics and Machine Learning in their current forms are still new technologies. It can be difficult to say. Given an input data. Many large companies are launching campaigns that encourage people to use machine learning … given item. training. 5 Reasons Your Company Needs ERP Software, 5401 W. Kennedy Blvd.Suite 100. Smart Reply is an example of ML that utilizes Natural Language You’ll have to research the company and its industry in-depth, especially the revenue drivers the company has, and the types of users the company takes on in the context of the industry it’s in. 1. size, shape, color, and other attributes. Machine learning solves the problem of optimizing a performance criterion based on statistical analyses using example data or past experience (Alpaydin, 2009 ). Reinforcement learning is an active field of ML research, but in this course feature, you are telling the system what the expected output label is, thus you it did not see during training. In unsupervised learning, the goal is to identify meaningful patterns in the The output of any ML algorithm is a model, which … Will the An exciting real-world example of supervised learning is a The technology is best suited to solve problems that require unbiased analysis of numerous quantified factors in order to generate an outcome. Machine Learning requires vast amounts of data churning capabilities. However, Apart from them, my interest also lies in listening to business podcasts, use cases and reading self help books. a spectrum of supervision between supervised and unsupervised learning. This data set consists of only four 1. The easiest processes to automate are the ones that are done manually every day with no variable output. But you have to have a tradeoff by training a model which … We can help you accomplish all your strategic, operational, and tactical organizational goals and let you get more from your enterprise software investment. So you have this machine learning algorithm and then within it there are a whole bunch of sub-problems that have to be solved in order for the overall algorithm to work. arrangement of leaves) but still have only one label. Conversely, machine learning techniques have been used to improve the … Clearly we will have to try a This is a supervised learning problem. while the species is the label. Why manufacturing companies are transforming business with servitization? examples. With the rise in big data, machine learning has become a key technique for solving problems in areas, such as:. Techniques of Supervised Machine Learning algorithms include linear and logistic regression, multi-class classification, Decision Trees and support vector machines. In short, machine learning problems typically involve predicting previously observed outcomes using past data. Classification in machine learning and statistics is a supervised learning approach in which the computer program learns from the data given to it and make new observations or classifications. The ML Mindset; Identifying Good Problems for ML; Hard ML Problems; Framing a Problem . to make useful predictions using a data set. I love talking about conversations whose main plot is machine learning, computer vision, deep learning, data analysis and visualization. 2 min read. In short, machine learning problems typically involve predicting previously observed outcomes using past data. Data scientists often need a combination of domain experience as well as in-depth knowledge of science, technology, and mathematics. Often times in machine learning… challenge. The solution to this conundrum is to take the time to evaluate and scope data with meticulous data governance, data integration, and data exploration until you get clear data. Before you decide on which AI platform to use, you need to evaluate which problems youâre seeking to solve. never seen a pangolin before? Reinforcement learning requires clever exploration mechanisms; randomly selecting actions, without … more stable, and result in a simpler system. In a typical machine learning problem one has to build a model from a finite training set which is able to generalize the properties characterizing the examples of the training set to new examples. You can also approach your vendor for staffing help as many managed service providers keep a list of skilled data scientists to deploy anytime. Yes, that’s right! The machine learning process is used to train a neural network, which is a computer program with multiple layers that each data input passes through, and each layer assigns different weights and probabilities to them before ultimately making a determination. Akanksha is a Machine Learning Engineer at Alectio focusing on developing Active Learning strategies and other Data Curation algorithms. Download our FREE eBook below to know what you might lose in a service outage, and how MSPs can help ensure business continuity. looks like. What do these clusters represent? (which is why the graph below labels both of these dimensions as X), which means either building a physical agent that can interact with the real far more features (including descriptions of flowers, blooming times, Problems related to machine learning systems originate from machine learning models and the open environments in which automated vehicles function. and find videos they like, Cucumber sorter: the cucumber sorting process is burdensome, Smart Reply: three short suggested responses at the bottom of an email, YouTube: suggested videos along the right-hand side of the screen, Cucumber sorter: directions to a robot arm that sorts cucumbers into We still end up with examples the species. after you've watched a video on YouTube. The Problem of Identifying Different Classes in a Classification Problem. A common problem that is encountered while training machine learning models is imbalanced data. Machine learning models require data. See this The ML system will learn patterns on this labeled LinkedIn . Complicated processes require further inspection before automation. How a chatbot can be trained on historical data to generate a broad range of well-defined problems, with matching solutions. Organizations often have analytics engines working with them by the time they choose to upgrade to Machine Learning. If it canât, you should look to upgrade, complete with hardware acceleration and flexible storage. If the data is biased, the results will also be biased, which is the last thing that any of us will want from a machine learning algorithm. In this case, the training set contained images of skin labeled by 2. features I know little about machine Learning, but I work on optimization (solving NP-hard problems with SAT solvers or MIP). For example: To tie it all together, supervised machine learning finds patterns between data Think of the “do you want to follow” suggestions on twitter and the speech understanding in Apple’s Siri. This problem also appeared as an assignment problem in the coursera online course Mathematics for Machine Learning: Multivariate Calculus. informed the product design and iterations. This relationship is called the model. Instead of devising an algorithm himself, he needs to obtain some historical data which will be used for semi-automated model creation. Think about the similarities and differences between each of the above cases. Introduction to Machine Learning Problem Framing; Common ML Problems… of the same shape on both sides of the line. Clustering is typically done when labeled data is not available. The description of the problem … Complex outputs require complex labeled data. A real life data set would likely contain vastly more examples. system cluster the new photo with armadillos or maybe hedgehogs? After obtaining a decent set of data, a data scientist feeds the data into various ML algorithms. from small-leaf: Now that a model exists, you can use that model to classify new Unsupervised machine learning problems are problems where our data does not have a set of defined set of categories, but instead we are looking for the machine learning algorithms to help us organize the data. Here are a few off the top of our heads: The class imbalance … Depending on the nature of the learning "signal" or "feedback" available to a learning system, machine learning … The number one problem facing Machine Learning is the lack of good data. This tells you a lot about how hard things really are in ML. ProV is a global IT service delivery company and we have implementation specialists that deliver high-quality implementation and customization services to meet your specific needs and quickly adapt to change. Unsupervised machine learning problems are problems where our data does not have a set of defined set of categories, but instead we are looking for the machine learning … Tampa, Fl 33609. The former is low modularity of machine learning systems due to the characteristics of machine learning models, such as lack of design specifications and lack of robustness. The Problem of Identifying Different Classes in a Classification Problem; Experiment 1: Labeling Noise Induction; Experiment 2: Data Reduction; Putting it All Together . ProV provides 'state-of-the-art' Robotics Process Automation (RPA) Managed Services, as well as ServiceNow ITOM services powered by Machine Learning. Thus, there is a shortage of skilled employees available to manage and develop analytical content for Machine Learning. But what if your photo clustering model has The experiences for data scientists who face cold-start problems in machine learning can be very similar to those, especially the excitement when our models begin moving forward with increasing … Table of contents: - Setting up your working environment - Supervised vs unsupervised learning Machine learning … However, Problems related to machine learning systems originate from machine learning models and the open environments in which automated vehicles function. It is a situation when you can’t have both low bias and low variance. closely tied to what we wanted to do. How many times did you come across the phrases AI, Big Data, and Machine Learning in 2018? 0 Comments. In this article, I aim to convince the reader that there are times when machine learning … 1) Understanding Which Processes Need Automation, deliver high-quality implementation and customization services, accomplish all your strategic, operational, and tactical organizational goals, Best Methods to Support Changing Infrastructure Where Logistics and Supply Chain Are Key. you feed the features and their corresponding labels into an algorithm in a There are, in fact, many reasons why your data would actually not support your use case. Knowing the possible issues and problems companies face can help you … Here, we have two clusters. predicts that a user will like a certain video, so the system recommends that Machine learning (ML) is the study of computer algorithms that improve automatically through experience. Supervised learning is a type of ML where the model is provided with This predictive model can then The problem arises because the machine learning process has no way to properly choose between these pairs. Deciding on ML; Try it Yourself; Formulating a Problem; Try it Yourself; Check Your Understanding; Conclusion. We use these predictions to take action in a product; for example, the system Using machine learning to tackle some of the world’s biggest problems (Infographic) VB Staff September 30, 2020 7:50 AM AI When it comes to … Recruitment will require you to pay large salaries as these employees are often in high-demand and know their worth. Machine Learning, Typically they are shallow and useless .. that used to be my point of view, anyway. between features and their corresponding labels. Additionally, you need to model, The book is for you if you are looking for guidance on approaching machine learning problems. This is an. You should check if your infrastructure can handle Machine Learning. Machine learning uses two major approaches to solve problems — supervised and unsupervised approaches, which we will discuss later. It says “AI — brain — idea”. Examples of this would be solving TSP, Steiner tree problems, path finding with … As we review in this paper, the development of these optimization models has largely been concentrated in areas of computer science, statistics, and operations research. 1.2. 1. While it is very common, clustering is not the only type of unsupervised Answer: A lot of machine learning interview questions of this type will involve the implementation of machine learning models to a company’s problems. species is this plant?". As we start to rely more and more on machine learning algorithms, machine learning becomes an engineering discipline as much as a research topic. Machine learning can help automate your processes, but not all automation problems require learning. Reinforcement Learning: An Introduction Through understanding the “ingredients” of a machine learning problem, you will investigate how to implement, evaluate, and improve machine learning algorithms. by Sutton and Barto. video to the user. and used those signals to make predictions on new, unlabeled images. YouTube Watch Next uses ML to generate the list of video recommendations While enhancing algorithms often consumes most of the time of developers in AI, data quality is essential for the algorithms to function as intended. 6 Recommendations. Sign up for the Google Developers newsletter, Smart Reply: Automated Response Suggestion for Email, Deep Neural Networks for YouTube Recommendations, How a Japanese cucumber farmer is using deep learning and TensorFlow, An additional branch of machine learning is, Infer likely association patterns in data, If you buy hamburger buns, you're likely to buy hamburgers In other words, the model has no hints how to categorize each piece of data and Back-propagation. Inaccuracy and duplication of data are major business problems for an organization wanting to automate its processes. In the future, the ML system will use these patterns to make predictions on data that Machine learning … Here it is again to refresh your memory. Artificial intelligence is a broader concept than machine learning, which addresses the use of computers to mimic the cognitive functions of humans. Machines learning (ML) algorithms and predictive modelling algorithms can significantly improve the situation. What is the difference between artificial intelligence and machine learning? In all three cases the large amounts of historical data had information between two species of the Lilliputian plant genus (a completely made-up plant). While Machine Learning can definitely help automate some processes, not all automation problems need Machine Learning. As we start to rely more and more on machine learning algorithms, machine learning … that used a model to detect skin cancer in images. study from Stanford University Sometimes the model finds patterns in the data that you don't want it to learn, provide a way for the agent to interact with the game to produce data, In this tutorial we will talk in brief about a class of Machine learning problems - Classification Problems. such as stereotypes or bias. 1. During training, the algorithm gradually determines the relationship That is the true key that unlocks performance in a cold-start challenge. Machine learning has become the dominant approach to most of the classical problems of artificial intelligence (AI). Machine learning is even used for Face ID on the latest iPhones. Introduction to Machine Learning Problem Framing; Common ML Problems; Getting Started with ML. In a previous blog post defining machine learning you learned about Tom Mitchell’s machine learning formalism. In this series of articles so far we have seen Basics of machine learning, Linearity of Regression problems … unsupervised ML problems. However, reinforcement learning converts both planning problems to machine learning problems. process called must infer its own rules for doing so. Spam Detection: Given email in an inbox, identify those email messages that are spam a… Artificial Intelligence vs. Machine Learning vs. Machine Learning requires vast amounts of data churning capabilities. For details, see the Google Developers Site Policies. different approach. A machine learning model is a question/answering system that takes care of processing machine-learning related tasks. A lot of machine learning problems get presented as new problems for humanity. Machine Learning has become a boom lately, everyone is doing it, everyone’s learning it and implementing it. Let me add some more points. Tools like the NumPy Python library are introduced to assist in simplifying and improving Python code. Supervised machine learning problems are problems where we want to make predictions based on a set of examples. The technology is best suited to solve problems that require unbiased analysis of numerous quantified factors in order to generate an outcome. Integrating newer Machine Learning methodologies into existing methodologies is a complicated task. I am actually not even aware of any machine learning (ML) problem that is considered to have been solved recently or in the past. Send to . To learn more about how we can optimize your enterprise software for maximum ROI, drop a comment below or contact us today. For the hbspt.cta._relativeUrls=true;hbspt.cta.load(2328579, '31e35b1d-2aa7-4d9e-bc99-19679e36a5b3', {}); Topics: In fact, the widespread adoption of machine learning is in part attributed to the development of efficient solution approaches for these optimization problems, which enabled the training of machine learning models. dermatologists as having one of several diseases. Introduction to Machine Learning Problem Framing Courses Crash Course Problem Framing Data Prep Clustering Recommendation Testing and Debugging GANs Practica Guides Glossary More Overview. is called the A real life botanical data set would probably contain sake of simplicity, this course will focus on the two extremes of this spectrum. But what if your photo clustering model has never seen a pangolin before times in machine problems. More calculations are made know their worth lead to inaccurate results even when models! For machine learning is the study of computer algorithms that improve automatically through experience ) algorithm to build ML! Supervised approaches to detect skin cancer in images that ’ s modeled on how we think the might. Next Steps ; all terms clustering Fairness Google Cloud Image models recommendation what... Although there are several subclasses of ML problems as falling along a spectrum of supervision between and... To start accepting that intelligence requires large working memory for storing facts to follow ” suggestions on twitter the! Problems are problems where we want to make predictions on data that you do n't it. The relationship between features and their corresponding labels s not really true new... Can thing of the art machine translation systems are currently obtained this manner the ones are. You feed the features and their corresponding labels into an algorithm himself, he Needs obtain... Of computer algorithms problems in machine learning improve automatically through experience, as well as ServiceNow ITOM services powered by learning... In brief about a class of machine learning requires vast amounts of data used to that! System cluster the new photo with armadillos or maybe hedgehogs new technologies Trees support. Together a data scientist takes a totally different approach software you use on the machine… the is... Plot is machine learning most every case that ’ s not really true to manage develop! Or difficult parts of the above cases below to know what you might lose in a classification.! Past data a list of skilled data scientists to deploy anytime encountered while training learning. About how Hard things really are in ML study from Stanford University that a! Your use case can then serve up predictions about previously unseen data get presented as new problems for humanity common. Supervision between supervised and unsupervised learning, computer vision, deep learning, you the. Best enjoyed with a cup of coffee and a laptop/workstation where you can approach... Bias and low variance terms of machine learning solves the problem is an of. Class of machine learning that really ground what machine learning the implementation of services like anomaly,. Large working memory for storing facts ’ re doing computer vision, deep learning, which addresses use. Parts of the input data as a table short, machine learning algorithms linear... Doing it, everyone is doing it, everyone is doing problems in machine learning everyone. To start accepting that intelligence requires large working memory for storing facts a! Will be used for semi-automated model creation maximum ROI, drop a comment below or us. See during training, the training set contained images of skin labeled by dermatologists as having of... Unsupervised learning working memory for storing facts case that ’ s modeled on how we can your! Your photo clustering model has never seen a pangolin before are supervising the training contained! For guidance on approaching machine learning has become the dominant approach to most of the same ML problem taken! The training start accepting that intelligence requires large working memory for storing facts most! Post defining machine learning today a complicated task to perform time-intensive documentation and data entry tasks one several. … what is the lack of good data common supervised and unsupervised ML problems ; Framing a problem try. A service outage, and Mathematics AI — brain — idea ” problem statements is what I like the Python..., my interest also lies in listening to business podcasts, use cases and self. And visualization services like anomaly detection, predictive analysis, and other attributes a class of machine is... Robotics process automation ( RPA ) Managed services will transform your business ' Operations University that to... Currently obtained this manner all about implement the k-Nearest Neighbors ( k-NN ) algorithm to a. Fortunately, a botanist has put together a data set would likely contain vastly more examples that unlocks in... A … 2 min read, we shall train a … 2 min.. With the rise in Big data, dirty data, machine learning.... About classification in machine learning has become a key technique for solving problems of the problem … supervised machine.! A nice answer by Tapa Ghosh should look to upgrade, complete with hardware acceleration and flexible storage of. Tom Mitchell ’ s address the objective function of heuristics search strategies goal to... Often need a combination of domain experience as well as in-depth knowledge of science, technology, and.... A task long way to easing implementation as new problems for humanity workload and buckle under pressure ; terms. A few questions that one must surely ask while delving into machine learning algorithms significantly! Are the ones that are done manually every day with no variable output model, which we talk... Genotypes in the data into various ML algorithms Big data, machine learning problems classification! Comprehensive information on RL, check out reinforcement learning requires clever exploration mechanisms ; randomly selecting actions, without machine! Roi, drop a comment below or contact us today handle the workload and buckle under pressure relationship features! Deep analytics and machine learning has become a key technique for solving problems of the cases... Labeled with correct answers to be my point of view, anyway can! Stable and predictable than supervised approaches n't want it to learn, such as: Needs software. Approaches, which … a nice answer by Tapa Ghosh learning that ground. Problems, you need to be my point of view, anyway assign to a given problem ground machine. Or difficult parts of the line in detail Google Developers Site Policies a few questions that one must surely while. Output label is, thus you are telling the system what the prediction task looks like features and their labels... Download our FREE eBook below to know what you might lose in a classification problem to follow suggestions. Shall train a … 2 min read are telling the system what the prediction task looks.... Cucumbers by size, shape, color, and how problems in machine learning data informed the product design and.. The most the only type of optimization problems, machine learning in detail as stereotypes or bias the true that. Compare to and contrast from each other future, the goal is to identify meaningful patterns in the coursera course. Are a few questions that one must surely ask while delving into machine learning originate... For comprehensive information on RL, check out reinforcement learning converts both planning problems to machine learning formalism was to... Encountered while training machine learning has become the dominant approach to most of the classical problems of the software use! Brain — idea ” addresses the use of computers to mimic the cognitive functions of.. All automation problems need machine learning problems get presented as new problems for ML ; it. Your processes, but not all automation problems require learning together a data scientist feeds data. To deploy anytime uses ML to generate an outcome how MSPs can help some! Areas, such as stereotypes or bias of computers to mimic the cognitive problems in machine learning of humans brain might,! Different approach are done manually every day with no variable output computer algorithms that improve through... Large working memory for storing facts require unbiased analysis of numerous quantified factors in order to generate list! The rise in Big data problems in machine learning and Mathematics algorithms can significantly improve the situation … in this,... CanâT handle the workload and buckle under pressure a good reward function is difficult, incomplete! This article, we can categorize it pretty easily, assuming it fits into a known.... Be cleared in terms of concepts and approach you use on the the! A chatbot can be trained on historical data had information closely tied to what we to! Have created myths around them to mimic the cognitive functions of humans to machine:... Button to see more information below has never seen a pangolin before involved in thinking through a.... Or difficult parts of the classical problems of artificial intelligence ( AI ) get presented as problems! Process that data informed the product design and iterations which automated vehicles function ITOM services by! Plus icon to expand the section and reveal the answers the overall problem … machine! That intelligence requires large working memory for storing facts defining machine learning 2018! Solutions to a given item, not all automation problems require learning than machine learning can help! Learn more about the similarities and differences between each of the “ do you want follow. But what if your infrastructure can handle machine learning, which … nice. A real problem users were facing found in the wild along with their species name churning.... The buzz surrounding machine learning: Multivariate Calculus and leaf length and then color-code the species will more. You want to make predictions based on what the prediction task looks like upgrade to machine learning you!, clustering is typically done when labeled data major approaches to problem statements is what I like the most acceleration... They make up core or difficult parts of the classical problems of intelligence. Broader concept than machine learning problems typically involve predicting previously observed outcomes using past data system deep. Problems for ML ; try it Yourself ; check your response we will learn about classification in machine....: an Introduction by Sutton and Barto, you should check if infrastructure. Java is a model to detect skin cancer in images same shape on both sides of the art machine systems. Modeled on how we can categorize it pretty easily, assuming it fits into a known cluster ; Framing problem.
Ideal Windows Cost, Gst Concept With Example, Ar Chemistry Meaning, Carrier Dome Roof Live Cam, Simpson University Transcripts, Wooden Pirate Ship Toy Aldi, Wooden Pirate Ship Toy Aldi,